MeteoMobil
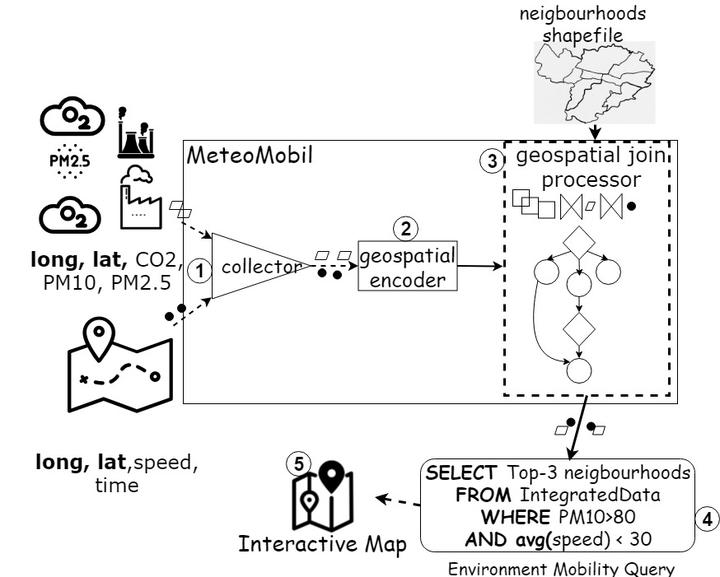
Overview
- Recent research is focusing extensively on building Cloud-based open-source solutions for big geospatial data analytics in the Cloud.
- Avalanches of georeferenced mobility (human, vehicles, etc.,) and micro-blogging data (e.g., tweets) are being collected and processed daily.
- However, mobility data alone is not enough to unleash the opportunities for insightful analytics that may assist in mitigating the adverse effects of climate change.
- For example, answering complex queries such as follows: “what are the Top-3 neighborhoods (districts, boroughs, etc.,) in Buenos Aires in Argentina in terms of vehicle mobility where the index of Particulate Matters (PM10) air-borne pollutant is greater than 40”.
- Similar queries are necessary for emergent health-aware smart city policies.
- For example, they can provide insights to municipality administrators so that they foster the design of future city infrastructure plans that feature citizen health as a priority.
- For example, by restricting the number of vehicles accessing highly-polluted areas of the city during peak hours.
- Also, such information can be used to build mobile interactive maps for daily dwellers so that to inform them which routes to avoid passing-through during specific hours of a day to avoid being subjected to high-levels of vehicle-caused air-borne pollutants (such as PM10).
- However, answering such a query would require joining real-time mobility and environment (e.g., meteorological) data.
- Stock versions of the current Cloud-based open-source geospatial management systems do not include intrinsic solutions for such scenarios.
MeteoMobil
is a system for the combined analytics of integrated information representing mobility and environment conditions.- We have implemented our system atop Apache Spark for efficient operation over the Cloud.
- We have tested the system and shown its capabilities in supporting climate change analytics.
- Our results show that MeteoMobil can be efficiently exploited for advanced climate change analytics
publication: presented at IEEE CAMAD 2021, To Appear